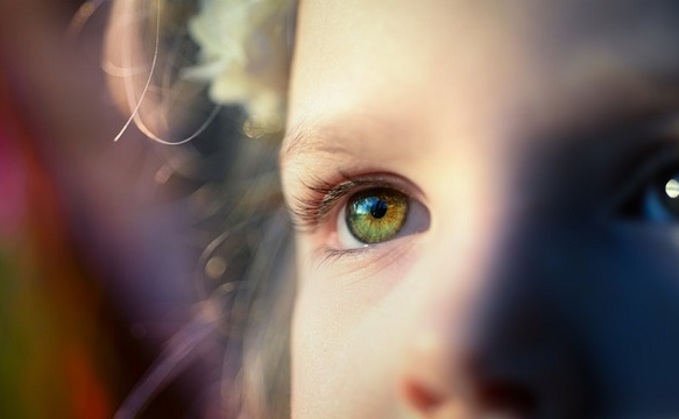
AI model achieves perfect accuracy in diagnosing childhood autism using retinal images
Researchers from Yonsei University College of Medicine in South Korea have successfully used AI technology to diagnose childhood autism with unprecedented accuracy.
The study, published in the journal JAMA Network Open, reveals that an AI algorithm, trained on retinal images, achieved a remarkable 100% accuracy in distinguishing children with autism spectrum disorder (ASD) from those with typical development.
This revolutionary approach not only promises a more accurate and earlier diagnosis but also addresses the challenge of limited access to specialised child psychiatry assessments.
ASD is a developmental condition believed to impact approximately 1 in 100 children worldwide. Its origins are considered to be influenced by a combination of genetic, social and environmental factors. The disorder is characterised by two primary features: challenges in social communication and a proclivity for engaging in repetitive behaviours, often without the typical capacity for behavioural flexibility.
According to the United States Centers for Disease Control and Prevention (CDC), ASD's prevalence within the country is estimated to be 1 in 36 individuals, and this number appears to be increasing annually.
Previous studies have established a connection between changes in retinal nerves and alterations in brain structures, ultimately linked to ASD.
"Individuals with ASD have structural retinal changes that potentially reflect brain alterations, including visual pathway abnormalities through embryonic and anatomic connections," the researchers noted in their paper.
The study, involving 958 children with an average age of 7.8 years, aimed to harness the potential of retinal photography and deep learning AI algorithms in ASD diagnosis.
The researchers captured a comprehensive dataset of 1,890 retinal images, half from children diagnosed with ASD and the other half serving as age- and sex-matched controls.
At the core of the research was a convolutional neural network, a deep learning model, trained on 85% of the retinal images and corresponding ASD symptom severity scores.
The remaining 15% of images were reserved for testing.
The AI model exhibited an unprecedented mean area under the receiver operating characteristic (AUROC) curve of 1.00 when screening for ASD. In simpler terms, the AI achieved a flawless 100% accuracy in distinguishing children with ASD from those with typical development (TD).
Remarkably, even when 95% of non-critical areas of the retinal images were removed, the AI's diagnostic performance showed no significant decline.
The AI's capabilities extended beyond mere diagnosis, showing promise in assessing symptom severity with a mean AUROC value of 0.74, falling within the "acceptable" range.
The study included children as young as four.
The researchers emphasise that their work represents a crucial step toward developing objective screening tools for ASD, offering hope for early and accurate diagnoses that could positively impact intervention and support strategies for affected children.
However, they highlight the need for further research to validate its accuracy for younger participants, considering that the retina of a newborn child continues to develop until the age of four.
"These findings suggest that retinal photographs may be a viable objective screening tool for ASD and possibly for symptom severity. Retinal photograph use may speed the ASD screening process, which may help improve accessibility to specialised child psychiatry assessments currently strained by limited resources," the researchers wrote.